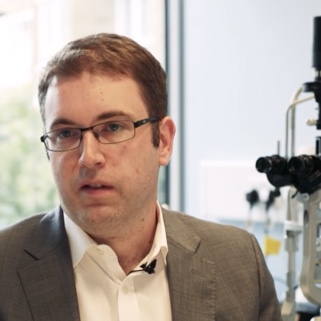
As part of our podcast series we spoke to Pearse Keane, retinal specialist at Moorfields Eye Hospital and a NIHR clinician scientist at the University College London Institute of Ophthalmology, who is talking in the Health Tech stream at the 2020 conference. Pearse shares his story about how he came to collaborate with DeepMind to use deep learning to identify age-related macular degeneration (AMD), a devastating but common retinal disease that, if not treated quickly enough, leads to blindness.
Why medicine?
I come from a medical family in Ireland, so it seemed like medicine was inevitable as a career path. I’d always been interested in physics and optics, and the biology of vision. I liked the idea of doing surgery that was done on the scale of millimetres, and could bring such profound benefits to patients.
Ophthalmology always seemed to be one of the most technology-driven of the medical specialties.
Ophthalmology always seemed to be one of the most technology-driven of the medical specialties. Even going back to the 50s, when lasers were being developed for the first time, some of the first medical uses of lasers were treating diabetic eye disease. And then more recently, getting rid of peoples glasses. You’ve also got the micro surgery, as I’ve just described.
Coming forward to the present day, ophthalmology has been at the forefront of gene therapy and stem cell therapy. What’s been exciting for me is that it’s been at the forefront of artificial intelligence as well. And I’ve been able to jump on board with that.
Collaborating with DeepMind
In 2016 you began a collaboration with DeepMind to use deep learning with OCT scans to identify age-related macular degeneration. Could you share the story behind your collaboration?
There’s probably two big overlapping things that led to this happening. The first is that in my role as a consultant, as a clinician – actually in the trenches treating people – I was getting frustrated with the challenges that we face in ophthalmology. For example, ophthalmology is one of the busiest of all the medical specialties. There’s nearly 10 million clinic appointments in the NHS just for eyes every year. And that’s nearly 10 percent of all outpatient appointments.
So we are drowning in the number of patients that we need to see. And so the result is that there are some people who are going blind because they can’t be seen and treated quickly enough. To capture that in one concrete example, there was a patient of mine called Elaine Manna who spoke publicly about her condition. She has age-related macular degeneration (AMD) and she lost sight in her left eye before there was good treatment for this condition. And then in 2012-2013, she started to lose sight in her good eye. AMD is the most common cause of blindness in the UK, Europe, the US, and in many other places.
She went to her local optician and the optician told her, I think you’re developing wet AMD, the severe form of this condition, in your good eye. But the good news is that we now have some treatments for this. We can give you injections into your eyes that can save your sight. You need to see a retinal specialist urgently to begin that treatment.
If that was my mother, I would want her to be seen and treated in six days, not in six weeks.
So she goes to her GP. She gets an appointment, but she gets an appointment back in the system, six weeks later. If that was my mother, I would want her to be seen and treated in six days, not in six weeks. As it happens, Elaine was able to get into Moorfields and get seen and treated in a timely fashion, and she’s been able to maintain her sight. But it was that sort of frustration that drove me.
Part of the reason why that’s difficult, is because for example in 2016 Moorfields had 7,000 urgent referrals as possible wet AMD. Of those 7,000 referrals only 800 of those actually had the condition. So a lot of the time what would happen is, you would have people who really didn’t have anything wrong with them, who are getting stressed thinking they were going blind. Often younger people push to get into the system quickly, and too often I would see older people who don’t want to cause any waves in the system, who would wait patiently for their appointment and then perhaps wait too long to be seen and treated.
My simple idea was to use the latest advances in AI, and in particular this technique that we call deep learning, to essentially run on these eye scans – which are called OCT scans or optical coherence tomography scans – to be able to prioritise those patients with the most eye threatening disease.
So my simple idea was to use the latest advances in AI, and in particular this technique that we call deep learning, to essentially run on these eye scans – which are called OCT scans or optical coherence tomography scans – to be able to prioritise those patients with the most eye threatening disease. So that they can get in front of someone like me within days instead of weeks or months. And the people who don’t have sight threatening disease, they would still be seen, but maybe they would be safer for them to wait a few weeks to be seen.
The second part of the story is that as an academic, I was hearing about all of the latest advances in deep learning in the tech world, I was reading stories in the New York Times about the “great AI awakening” and all the hype around AI, and how it’s transforming the tech world. I was thinking, how do I get collaborators who have deep learning expertise to work with?
Initially I was looking for some academic collaborators. And to be honest I was met with shoulder shrugs to a large extent at that point. Maybe that was because I was also at a more junior point in my career as well, or multiple different reasons. But I then started to think, what about industry? Because at the time, which was maybe 2014-2015, there was this idea that to do the latest deep learning you needed to have a lot of computing resources, and you need to have really specialised technical expertise. And a lot of that was residing in the big tech companies.
I read a profile in Wired UK magazine on DeepMind in July 2015. DeepMind had a lot of excitement about them, but what struck me was that two of the three co-founders of DeepMind were graduates of UCL, which is the University affiliated with Moorfields.
I was thinking, who do I contact? If I send an email to Bill Gates, that’s probably too much. But if you send an email to someone who’s very low down in an organisation that’s not going to work either. So I read a profile in Wired UK magazine on DeepMind in July 2015. DeepMind had a lot of excitement about them, but what struck me was that two of the three co-founders of DeepMind were graduates of UCL, which is the University affiliated with Moorfields. Two of the three co-founders were from London.
In fact, part of the interview is with Mustafa Suleyman, who used to head the applied division of DeepMind. And in this article it said something like, when they were being acquired or invested in they had been pressured to move to Silicon Valley, and that they actually wanted to stay in London. I found Mustafa Suleyman’s LinkedIn profile and asked him whether we could work together to apply deep learning to OCT scans, to prioritise those people with sight threatening disease.
To my joy and excitement, he sent me a message shortly after. And a few days later I was in his office chatting to him. And so, here we are now five years later and on this journey to make things happen.
Clinical trials: Proof of concept
Nature Magazine published a paper about the work you and DeepMind have been doing developing a deep learning system for future prediction of wet age-related macular degeneration. So can you share with us the findings, and their significance.
We published two papers in Nature Medicine. The first paper was published in August 2018, after more than two or three years of work. That was the first paper that showed a proof of concept, that essentially we had trained a deep learning system that could look at retinal OCT scans and could assess those scans from more than 50 different retinal diseases. So not just macular degeneration. But also things like diabetic eye disease.
The performance which was on par with world-leading retinal experts at Moorfields, in terms of assessing those scans.
The performance which was on par with world-leading retinal experts at Moorfields, in terms of assessing those scans. With AI we always have to be careful because whenever you hear the hype around AI, often when you drill into the details, there’s a lot of caveats there. So this was a retrospective study that was done, but nonetheless, very very exciting.
The second paper that came out more recently was inspired by Elaine Manna, my patient. We investigated whether we can use deep learning as an early warning system for AMD, and in particular, can we predict the imminent development of wet AMD in the good eye. Because that’s what patients are really worried about.
The paper has shown proof of concept that we can do that. And so where we are now is that we’ve been working to see how we can go from a research paper to actually implementing this at scale in the real world. I thought that getting the research paper was the challenging part, but in actual fact that’s the easy part compared to actually making it happen in the real world. We’re now beginning to think about how we can use this early warning system for AMD in future clinical trials.
The challenges of going from theory to practice
If I think back to a few years ago as a clinical academic, I was very naive. I don’t have a computer science or engineering background. So I thought, you make an algorithm, you show it in a paper, and you’re good to go. But it turns out that’s not true at all. The piece of experimental code that is used in a research paper is in no way ready to be used in the real world.
The analogy that I would use is, it’s like you’ve built a concept car, and you’ve used that prototype car to break a world land speed land record. You have one or two goes, you break the world record, but there’s a team of engineers and scientists who are ready to tweak every part of it. And that’s a world of difference from having a car that actually can be manufactured in millions, and shipped all around the world, and safely deployed.
Although we’re excited about the potential of AI, we’re also appropriately cautious. Because there’s lots of ways that it won’t work, and there’s lots of dangers and opportunities for bias.
To more directly answer your question, we’re trying to make sure that what we’ve done in this first paper is clinically valid. Because although we’re excited about the potential of AI, we’re also appropriately cautious. Because there’s lots of ways that it won’t work, and there’s lots of dangers and opportunities for bias. For example, if it works in patients coming to Moorfields, will it work in different parts of the UK, or in different parts of the world, or in slightly different disease presentations. There’s lots of care required in that regard.
We don’t want to actually use anything in the real world and with patients until we really properly validated it. But then secondly, there’s the technical maturity of the algorithm. It has to be rewritten essentially so that it can run in a fraction of the time, with a fraction of the computing power. And all of the kinds of industrial goods manufacturing practices that are required, so that you can then get FDA approval or CE marking and all of those things. Those are the things that are taking a long time now.
Bias in eye scans
What we do know is that different eye conditions affect, for example, men and women differently. They affect people of different ages differently, and affect different ethnic groups in different ways.
Regarding bias in eye scans, there’s the known unknowns and then there’s the unknown unknowns. So what we do know is that different eye conditions affect, for example, men and women differently. They affect people of different ages differently, and affect different ethnic groups in different ways.
In our paper in Nature Medicine that came out in May, one of the things that we’re proud of is that if we look at the ethnicity make up of that paper, it reflects the fact that the data is from Moorfields, which is very diverse. And in fact, in that data set of patients with AMD, it is 55% white British or Irish, It’s 10% Asian, 33% other, and 2% Black. That’s very good compared to the main clinical trials of AMD. The big trials were 98 or 99% white.
Now having said that, and before we get too carried away patting ourselves on the back, the fact is that it’s 2% Black as an ethnic group in our in our city. Now that reflects the fact that people who have more pigmented skins don’t tend to get macular degeneration as much as people with fair skin. That’s just the nature of the disease. On the other hand if you’re one of the few people of, for example, African origin, who has macular degeneration, you wouldn’t necessarily be too reassured to see that the algorithm has only been trained on 2% people like me.
One of the things that we’re doing now, is global validation studies. We’re trying to work with partners from every different continent to show that the algorithm will work as well in those different locations.
So one of the things that we’re doing now, is global validation studies. We’re trying to work with partners from every different continent to show that the algorithm will work as well in those different locations. And if it doesn’t work as well, to identify that at an early stage. Then we can augment the training data with additional information to make it more robust in that regard.
Collaborate to innovate
I think that this brings me to a broader point. Which is that to really drive innovation, you need to have collaborations that are at the boundaries between different disciplines. Whether that be clinicians, computer scientists, anthropologists, or design – you name it – the exciting stuff happens at the boundaries between different disciplines. And that’s I think part of the reason why I’m so excited to speak at and attend this conference.
I think innovation has to occur at the boundary between academia and industry. What I’ve come to learn is that, the idea that an academic could somehow train a clinical AI system and then have that used by millions of people around the world is completely naive. It would require significant industry involvement.
But also, the other thing is that I think innovation has to occur at the boundary between academia and industry. What I’ve come to learn is that the idea that an academic could somehow train a clinical AI system and then have that used by millions of people around the world is completely naive. It would require significant industry involvement. Now of course, that could be with a multinational tech company like Google, or it could be with a startup, or it could be with a spinout, or something like that.
And of course there are pros and cons to each, but ultimately you have to work with industry if you’re going to have a commercial product that can bring benefits to patients. And the way I view it is, I’m driven by patient benefit. Patient benefit is our North Star, and then see what we need to do to work backwards from there to make that happen.
UKRI Future Leaders Fellowship
Earlier this year you were awarded a UKRI Future Leaders Fellowship. As a Future Leaders Fellow you’re being funded for work involving the development and application of AI in the NHS. Can you tell us what that funding means to you and your work?
I went to medical school in Ireland and did my training there, and I went to Los Angeles for a couple of years. And my idea when I went to the States was that I would stay there long-term. Because I felt that that was a place which had very good opportunities for clinical academics. By chance I met an Irish guy who was working in Moorfields. He told me about the National Institute for Health Research (NIHR) in the UK, and he said that there’s this funding scheme that allows you to have protected time for research and for clinical time. And long story short, almost there and then, I decided that I would come to Moorfields, come to London if they’d have me, and get this NIHR funding.
This is seven years of funding that’s specifically aimed at ambitious, adventurous long-term projects that are about impact, innovation, and getting stuff done.
I was really lucky to be funded by the NIHR for a number of years, and my funding ended at the start of this year. And the exciting thing was that there was this new funding scheme from the UK Research and Innovation, a Future Leaders Fellowship. And this is seven years of funding that’s specifically aimed at ambitious, adventurous long-term projects that are about impact, innovation, and getting stuff done. So I put together this application which I gave the rather grand title of, “Enabling the Development and Application of Artificial Intelligence in the NHS”.
And the idea behind that was we could take what we’ve learned in ophthalmology and continue to translate that. But then use that as an exemplar for other medical specialties, of how to develop and apply AI in the NHS. And that’s not just on the technical and the scientific side, but also on the more difficult questions related to ethics, data protection, and transparency. Because ultimately these AI systems all involve the training of the models using clinical NHS data, and that has to be handled very sensitively and done appropriately.
What I wanted to do is share our learnings from that, just to try and improve these efforts because of the pressing need. But also, just this idea that if we get our act together, the NHS and the UK can be world leaders in this space.
Three challenges to overcome for AI in medicine
Your work has highlighted three major challenges that must be overcome if AI is to be rolled out more quickly in medicine and elsewhere. The first was getting data into coherent usable format. The second was privacy in regulation. And the third was explainability. Can you touch on each of those?
There’s something that I’ve heard referred to as ‘hearsay data’. I think it might be Neil Lawrence from Cambridge who used this term. Hearsay data is that everybody says, I’ve got the world’s biggest data set of X. All the time I’m getting approached by consultants and clinical researchers and they say, I’ve got the world’s biggest data set of X-rays, or condition X.
It turns out that they tell you that they’ve got 10 million scans. Which means they only have 1 million scans. But actually, of those 1 million, the controllers for that are like five different institutions. And at their institution, they only have a hundred thousand. And of those hundred thousand, they’re in 10 different file formats. And of the file formats you’re interested in, they’ve only got labels for 10 percent of those. And the labels are only accurate in 10 percent of those.
The challenge has been getting really good large data sets with the right labels for them, to drive these advances in deep learning.
So you started with the 10 million scans, and quickly you get them to like 100 scans or 1000 scans. The challenge has been getting really good large data sets with the right labels for them, to drive these advances in deep learning. Because at the moment most of the advances in AI are driven by supervised learning, which is where you have labels for the data sets. That’s the first thing.
There’s no easy way to overcome this challenge. It would be very common when people approach me, or when I’m sat in at meetings at DeepMind for example, that they get approached by a clinician who says, we’ve got this data set. And they’re really excited and enthusiastic, because there’s some pressing clinical needs.
We would say, can you go away and find out more granular detail about the numbers of scans, the different file formats, who and which institutions are involved, what approvals are in place, and all of those things. And those enthusiastic clinicians typically say at the end of the meeting, I can’t wait to work on this. And then you never hear from them again.
Because it’s really difficult to find out the answers to that. Because oftentimes it may involve interacting with the IT department of your NHS trust, and the IT department are busy enough with just keeping the show on the road without somebody asking about these random research questions. There isn’t any clear roadmap yet about how you figure out all the issues around changing the file formats of data sets. How you figure out the governance. How you figure out the ethics.
On the one hand I had this attitude of, my patients are going blind, let’s not mess around here. But also realising that this is something that deserves caution, scrutiny, and transparency.
I guess that feeds into the next issue, which is sensitivities around data use. For example, in my case, I had initiated the collaboration of DeepMind because of this pressing clinical need. And because there were all of these nice coincidences that seemed to suggest that they were the good people to approach. But at the same time, DeepMind are a company that are owned by Google. And that’s a multinational tech company. And I think appropriately, there are sensitivities about that. On the one hand I had this attitude of, my patients are going blind, let’s not mess around here. But also realising that this is something that deserves caution, scrutiny, and transparency.
So one of the ways that we’ve tried to address that, is that from the very start we wanted to tell people what we’re doing, and engage as widely as we could. So for example, there’s a part of the Moorfield’s website which is dedicated to the collaboration with DeepMind. There’s FAQs, and there’s videos, and it has information if people want to opt out of the research, and things like that. When you go to Moorfields, there’s the TVs in the waiting rooms, and one of the screen savers that flashes up from time to time that says, Moorfields has this collaboration with Google, if you want to learn more, or if you want to opt out please get in contact. There’s posters up all around the hospital.
One of the nice aspects of the collaboration is that it got it a lot of media attention as well. I think that’s why the UKRI Fellowship was a good opportunity to try to share the learnings of what we did.
When we announced the collaboration, we also brought on board the Royal College of Opthamologists. We also got all of the major eye disease charities, the Macular Society, the Royal National Institute of Blind People, Action Against AMD, and the like. And we also got patient groups. So we combined that with lots of public engagement. One of the nice aspects of the collaboration is that it got it a lot of media attention as well. I think that’s why the UKRI Fellowship was a good opportunity to try to share the learnings of what we did.
We also published our research protocol in an open-source journal back in 2016 before we had done any scientific experiments whatsoever. Like I say, this was about telling people what we’ve done, but also looking for constructive criticism about what we’ve done. Because at the end of the day, we want to ensure patient benefit, but if there are ways that we can do this better, then we’re open to feedback.
Of course, we went through all the research ethics approvals required from the NHS Health Research Authority. I’ve given a number of talks related to to the project to the chairs of every research ethics committee in the country. And at the All Staff Day of the Health Research Authority, to tell them what we’ve done. There’s actually now a small section of the HRA website, where there’s a little video where I’m interviewed. One thing that I’m proud of is that the banner headline on the paper, where I highlight the importance of patient and public involvement and transparency.
Appreciating that there’s tons of hype with AI and that I’m contributing to it in no small part, I do think it has the potential to transform healthcare, and I do think it will become pervasive in the coming years. So we might as well share best practice as widely as we can.
If you’re doing the right thing for the right reasons, and there’s a clear patient benefit from what you’re doing, then I think most people are very supportive of this sort of work. Appreciating that there’s tons of hype with AI and that I’m contributing to it in no small part, I do think it has the potential to transform healthcare, and I do think it will become pervasive in the coming years. So we might as well share best practice as widely as we can.
Privacy and regulation
The privacy and regulation relates a little bit to the sharing. Because the data sets that we share – these anonymised, retrospective OCT scans – we’ve basically stripped out any identifiable data. I think that that’s important to recognise, because one of the pledges of the NHS Constitution from 2011 is that retrospective anonymised data should be used for the benefit of all patients on the NHS.
There is at least a theoretical possibility that patients could be re-identified from these scans. So basically what we’ve tried to do is ask ourselves, how can we take every possible measure to anonymise the scans?
The tricky part is that we anonymise the data to the standards specified by the Information Commissioner’s Office and beyond. But the fact of the matter is, that there is at least a theoretical possibility that patients could be re-identified from these scans. So basically what we’ve tried to do is ask ourselves, how can we take every possible measure to anonymise the scans?
For example, as well as just removing the name, the date of birth, the hospital number, the NHS number and things like that, we also take other measures. If we only have one patient that’s 114 years old, we don’t give that patient’s age. We say, greater than 90 years, or something like that.
We try to reduce the risk of re-identification by bucketing the data. Now even doing that, there could be a risk. So the next question is, want additional safeguards can we put in place?
Or suppose we have a retinal disease, and there’s less than a certain number of cases in Moorfields. Maybe there’s one, maybe there’s five, or something like that. We say, we’re not going to share that. Because imagine you’re the only person in the world with this condition. That identifies you as having this. So for every bit of clinical metadata we share we weigh up those. We try to reduce the risk of re-identification by bucketing the data. Now even doing that, there could be a risk.
The next question is, want additional safeguards can we put in place? One of the things we try to do is data minimisation. So we say, what’s the data that we need to use to address this problem. And interestingly, a few years ago, we would have thought that something like ethnicity is maybe not necessary. But actually we’ve now realised that that’s crucial.
You might say, what good is that? But I think that there is some additional reassurance in that. Because if a company like Google were [to breach the contract and try to re-identify the data], under GDPR now there are significant fines that could be leveraged against them.
We minimise the data, but then we also say, what other safeguards can we put in place? So for example, as part of the collaboration between Moorfields and DeepMind we put contractual safeguards. So for example, DeepMind or Google are not allowed contractually to link the data with other data sets. And they are not allowed to attempt to re-identify the data. Now, you might say, what good is that? But I think that there is some additional reassurance in that. Because if a company like Google were to do something like that, under GDPR now there are significant fines that could be leveraged against them.
The next thing is, what is the potential harm that could come if that tiny risk of re-identification happens? And in our opinion the harm that could come from re-identifying an old OCT scan is small compared to the harm that’s coming from all these patients going blind from not being seen and treated. So we have already talked about the crucial part, which is just being transparent. Simply telling people that this is what we’ve done. These are the safeguards we put in place. What do you think of this? How can we improve it? And then go from there.
Working together
Could you speak more on being a clinician and academic, but also working in Industry?
I read this book recently called The Innovators by Walter Isaacson. It was fascinating. There’s a section where he talks about this guy called Vannevar Bush, who was the head of MIT in the 1940s in the US. Because of World War II, he became director of research for the US government.
And essentially, he brought together government, academia, and industry. And that led to a golden era of digital innovation in the US. And it just occurs to me that now the world is going through some similar convulsions with the coronavirus pandemic. And so maybe this is another time to consider how we can get stuff done by working together.
You can listen to the full interview with Pearse on our podcast here.
Find Pearse on Twitter